The Advantages of Virtual Barrier Video Analytics for Perimeter Systems
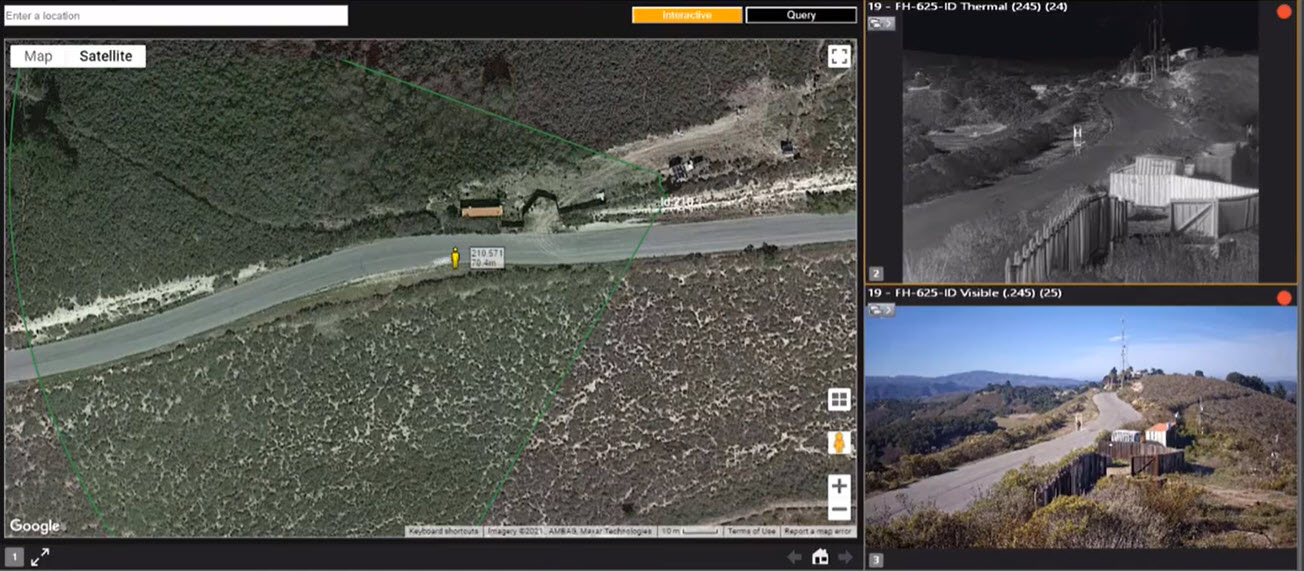
Artificial intelligence (AI) and machine learning have transformed the perimeter security landscape through the use of video analytics for intrusion detection. Highly-sensitive motion-based analytics, capable of detecting microscale movement at impressive ranges, have seen widespread adoption across perimeter systems deployed at critical infrastructure sites. Today, however, FLIR Virtual Barrier video analytics are yielding even greater benefits for customers, improving detection precision, classification accuracy, geolocation of targets and resilience against false alarms. This tech note will discuss the strategic value of Virtual Barrier analytics in perimeter intrusion detection systems (PIDS) as well as its specific performance advantages when compared to motion-based analytics.
Defining Motion-Based Analytics
Running video analytics with analog-based surveillance systems created a paradigm shift in the security industry in the early 2000s. Initial pixel-based motion detection, which triggered alerts based on a percentage of pixels that changed on a screen within defined borders, were able to run motion detection algorithms using onboard hardware. This allowed systems to deliver detection alerts instantaneously and operate on the edge while avoiding any bandwidth or latency issues.
Figure 1: Examples of humans being detected and classified by video analytics
These analytics operated on algorithms consisting of three steps: Background Initiation, Foreground Detection, and Foreground Processing.
The first creates a reference frame by building the background based on previous images. The second compared the immediate frame to the backgrounded frame, concluding that any difference between the two must be motion. Within the third step, the pixels flagged to be in motion are filtered to remove non-relevant causes and processed to generate intrusion alarms. While these motion-based methods can be highly sensitive when detecting threats, they also tend to require significant calibration and configuration to minimize false alarms in real-world environments. Even when calibrated properly, some environments still generate false alarms with motion-based analytics. Noise motion—e.g., shaking trees, shaking camera, shadows, or reflections—cause most of these false alarms. Another issue involves objects that stand still for a time or appear stationary when moving along a camera’s axis at long range. These targets are subsequently absorbed into the background image and rendered undetectable. These limitations with motion-based analytics technology can be costly to security personnel and set the stage for the next generation of video analytics using neural networks.
Defining CNN Video Analytics
Virtual Barrier video analytics are based on convolutional neural networks (CNN). This video analytic technique is designed to replicate neurobiological systems and is able to find and classify objects in an image.
Conventional systems rely on motion detection to identify where a potential object is.
Any moving target is processed by a set of filters, which determine whether the object is a threat. It is, however, impossible to account for every single situation in the real world— which means that some level of false alarms are always possible. The CNN video analytics used by Virtual Barrier addresses this by allowing the algorithm to automatically determine which features and filters are the most relevant to find and identify a desired object. Creating such a system starts with manually analyzing tens of thousands of images to determine the location and classification of objects of interest. These images are then used to teach the neural network in an iterative process. Contrary to other CNN driven systems, Virtual Barrier analytics don’t use images from publicly available datasets, which are frequently employed by other systems. Instead, it only uses Teledyne FLIR datasets, which are uniquely targeting the security application. This not only further reduces false alarms, but also assures correct detection of all potential threats.
Figure 2: Virtual Barrier analytics classifying two people and determining their location and speed
Comparing Virtual Barrier and Motion-Based Analytics
The differences between Virtual Barrier analytics and motion-based analytics are important to understand when choosing between the two models. Virtual Barrier analytics are simple to calibrate and provide robust detection with minimal false alarms while also supporting loitering detection and geo-location of threats for visualization on a dynamic map. Motion-based analytics tend to offer longer detection ranges than Virtual Barrier analytics but are more susceptible to false alarms. The following is a breakdown of the distinct benefits Virtual Barrier analytics can add to your security system.
Robust Classification
Backed by a library of thousands of images containing important augmentations and variations in the visual presentation of detectable objects, Virtual Barrier analytics are trained to classify objects in real-world situations where targets may be slightly obscured or challenging to identify. The FLIR FH-Series ID, for example, detected 15% more threats than motion-based analytics in a sample of 100 unique scenarios.
Regarding classification range, motion-based and Virtual Barrier analytics present a tradeoff worth noting. Analytics require more pixels on target than motion-based analytics, so the classification range is reduced for Virtual Barrier analytics. The classification range for the FLIR FH-Series ID is reduced by approximately 20% compared to the FLIR FC-Series ID.
False Alarm Reduction
Figure 3: Virtual Barrier analytics classifying humans and vehicles
One of the top advantages for Virtual Barrier analytics is the reduction of false alarms. Because Virtual Barrier analytics do not use motion as an input for detection, they are much less prone to alarming on common sources of noise such as swaying foliage, camera shaking in the wind, and wild animals.
In fact, these analytics have been shown to reduce false alarms by 60% in a sample of 100 unique scenarios, which included noise related to extreme weather, animals wandering into frame, camera shaking, etc. This is a key advantage of Virtual Barrier analytics since false alarms are one of the costliest operational issues that security personnel experience today.
Geo-location for Target Tracking
Figure 4: Human target being accurately mapped using the geo-location feature of the Virtual Barrier analytics
FLIR Virtual Barrier analytics support geo-location of targets in a scene. This means that each target’s position, speed and heading are identified by the analytics and streamed as metadata to be used by a video management software (VMS) or other downstream software. Geo-location data can be seamlessly visualized on a dynamic map as shown in Figure 4, to provide security operators with situational awareness of threats near their facility. The geo-location data provided by the analytics are also effective for positioning a PTZ camera for more in-depth assessment of a target.
Detects Loitering Objects
Unlike motion-based analytics, Virtual Barrier analytics models support loitering detection functionalities and can detect and classify objects in frame, whether they are moving or not. Because motion-based analytics are unable to detect targets unless they move, these models offer a unique advantage over background subtraction-based analytics.
Designed for the Future
Designed for continuous evolution, Virtual Barrier analytics will continue to improve, able to address the intrusion needs of today and tomorrow for security personnel in critical infrastructure. Teledyne FLIR is committed to expanding libraries of images for ever-improving classification accuracy, as well as adding features to the analytics for field upgrades. Security directors can feel confident deploying cameras with embedded FLIR Virtual Barrier analytics as a solution for their current and future needs.
Key Takeaways
Virtual Barrier analytics are purpose-built to enhance threat detection, delivering greater accuracy and critical situational awareness when tracking and responding to intruders, while minimizing false alarms. Designed to continuously improve, these analytics are built to keep pace with today’s evolving perimeter technologies and threats. Contact your local Teledyne FLIR representative today to learn how Virtual Barrier analytics can strengthen your perimeter security.